Recent Content
Tags
Months
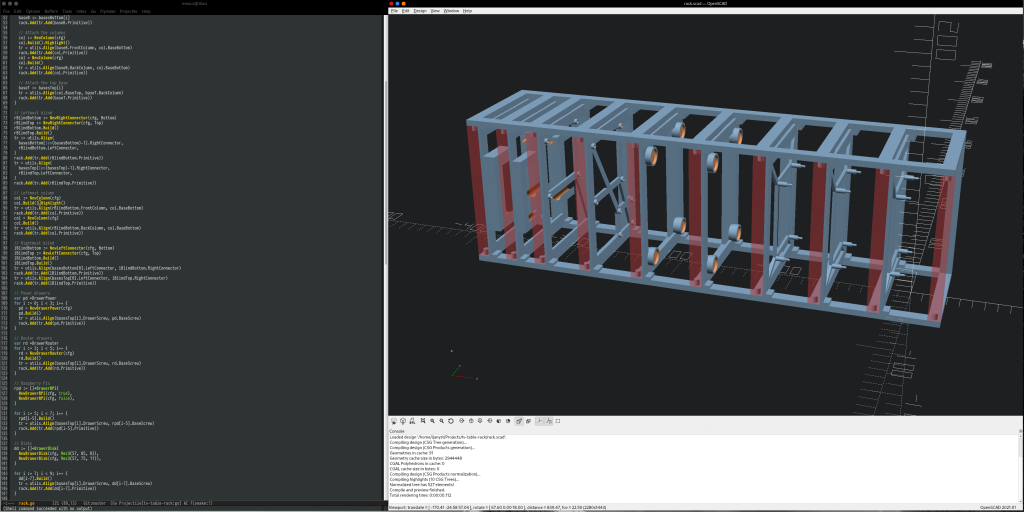
OpenSCAD is awesome. It gives you the ability to code up your 3D models and keep them readably under version control. But, despite the coolness, OpenSCAD has a bunch of limitations. This post introduces a system called GhostSCAD as a way around some of them.
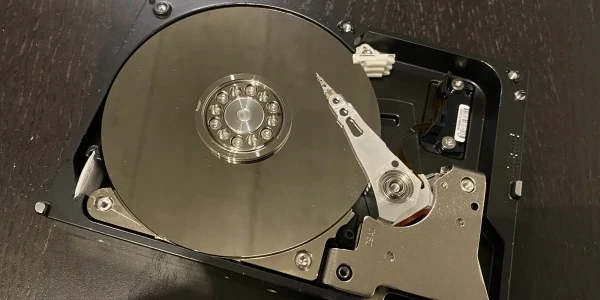
The NAS odyssey continues. The disks work, and we need to put them together in a reasonably optimal RAID array way. It necessitates some play with low-level formatting, block and stripe sizes of the RAID, and making sure that the filesystem settings don't mess everything up.
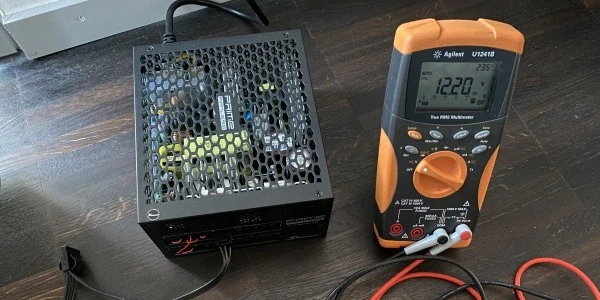
We have an ARM board that will run the show, and we need to have the bootloader, the trusted firmware, and Linux run on it. Once Linux runs, the disks need to spin when needed, shut up when not, and the transition between these states must be glitch-free. This post describes how to make that happen.
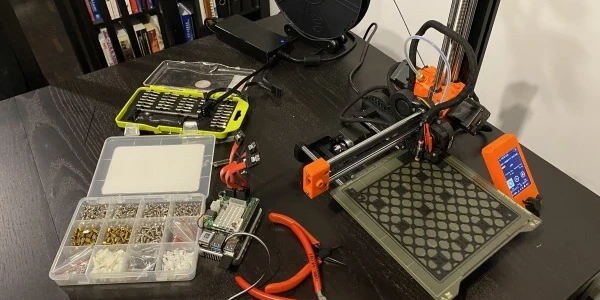
I need a NAS for the same reason that everyone needs a NAS. Unfortunately, I don't have a server room, so I need one that does not make any noise when idle. I could not find one, so I decided to build it. This post contains the bill of materials and a walkthrough the design and 3d printing of a NAS chassis.
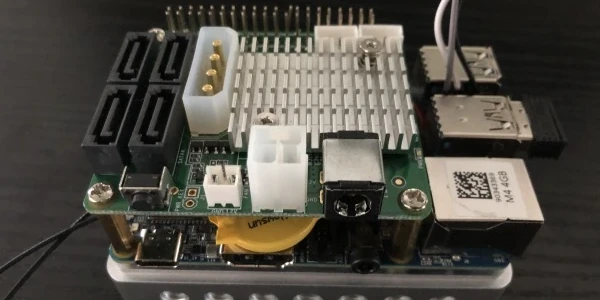
There are many pretty great ARM boards out there, but the stock firmware for most of them is ridden with binary blobs and is generally an abandonware. Fortunately, it's usually not that hard to make Debian work on most of them, including all the necessary multimedia peripherals.
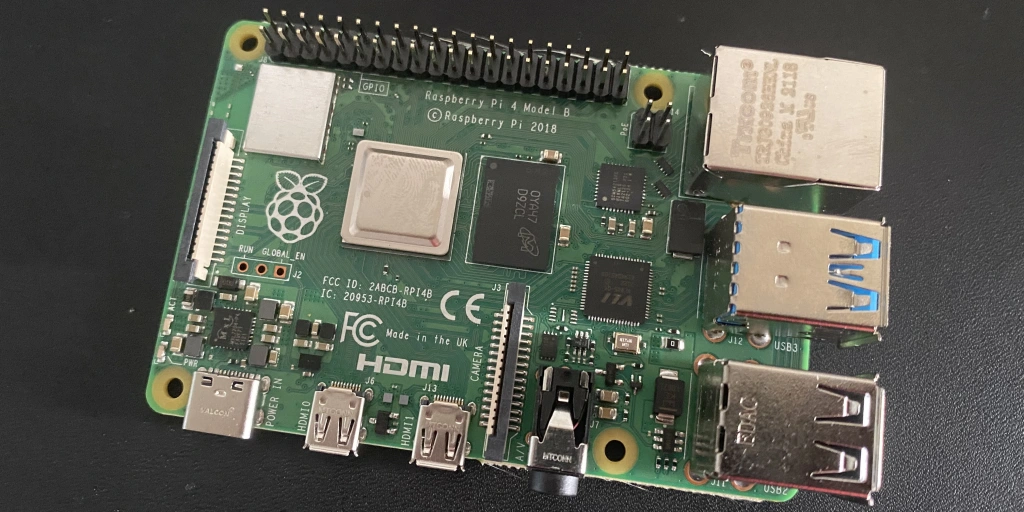
It's been quite a long while since the 64-bit CPUs took over the world. Most of the ecosystem moved on, but some of it didn't. The official OS for the RaspberryPi is one of the laggards, and it has started to be a problem. This post describes how to install the arm64 flavor of Debian on the device.
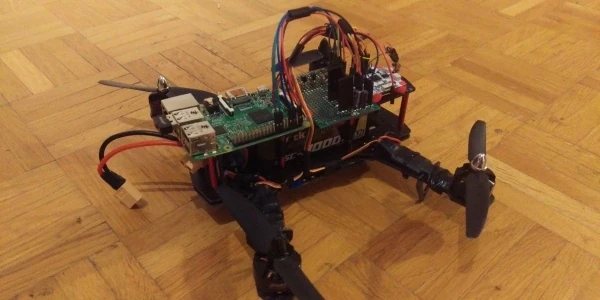
Ever since I can remember, I have wanted to build a quadcopter. Sure, you can buy one that will be way better than whatever you can make yourself, but where's the fun in that? The proper way to do it is to order a bunch of stuff that seems reasonable, put it together, make it (barely) fly, and iterate until the point where a swarm of them can play ping-pong.
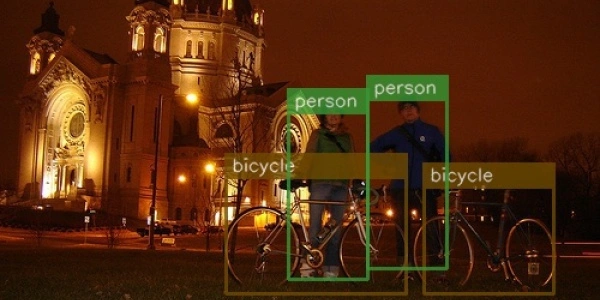
Some time ago, I wrote a post on building TensorFlow on a Jetson TX1. It worked eventually, but it was relatively slow because it's an edge device and it's not very powerful. It's way faster to compile it on a PC and copy the binaries to the target board. Here's how.
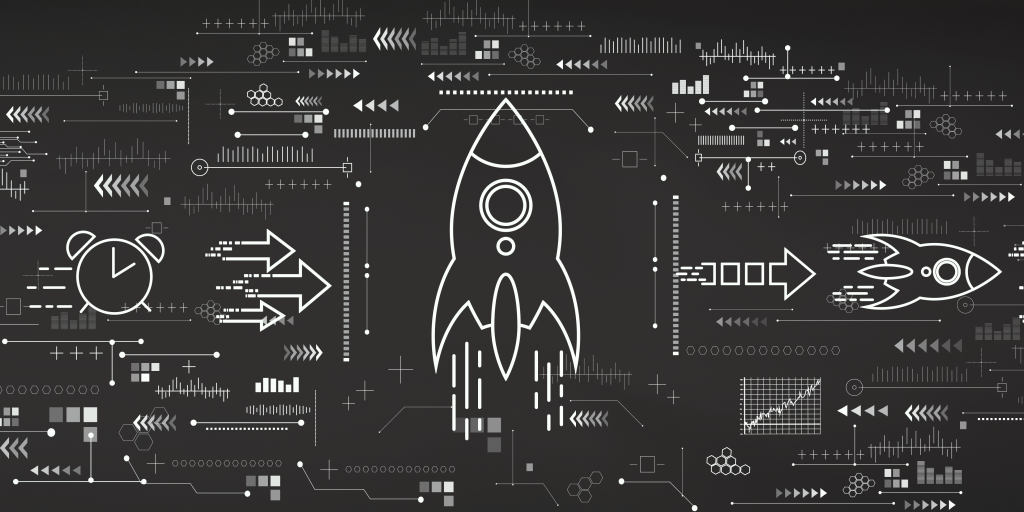
AWS has recently introduced the P3 instances. They come with Tesla V100 GPUs, so I decided to run a little benchmark to see how well they perform compared to my workstation (GeForce 1080 Ti) when training neural networks.
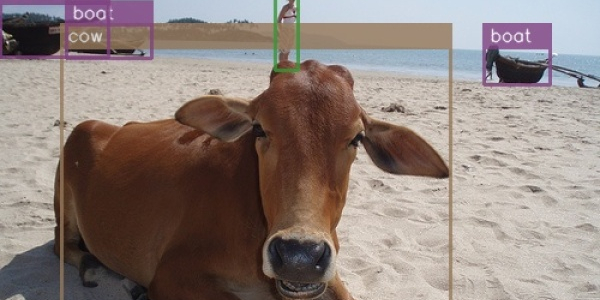
There was no understandable and straightforward implementation of SSD in TensorFlow, so I decided to make one. The original paper assumes familiarity with related research, so I needed to plow through several additional papers and a ton of source code to understand what's going on here. This post is an attempt to provide all that missing context in one place.