Recent Content
Tags
Months
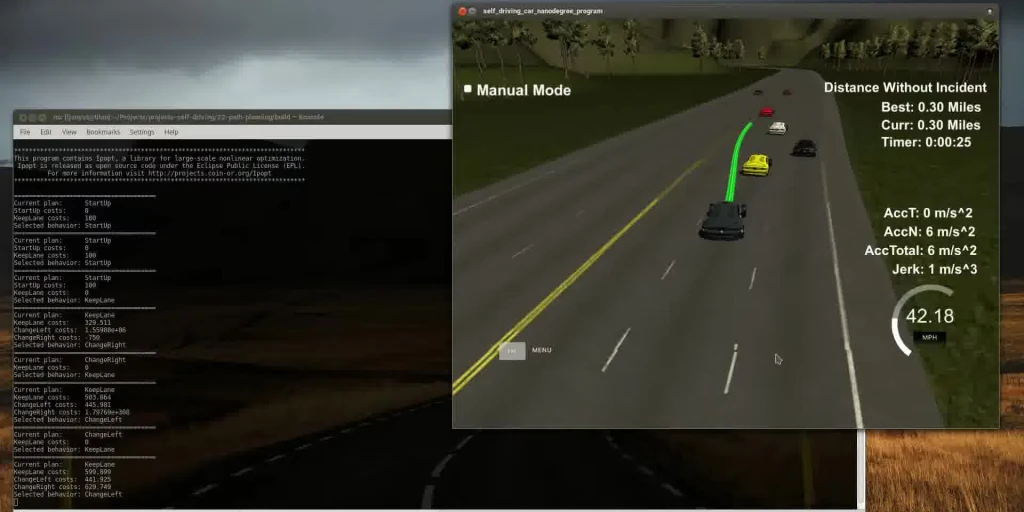
This post describes another one of the Udacity Self-driving Car Nanodegree projects. The goal is to plan a path or a car through highway traffic. You have to obey the rules, pay attention not to collide with anyone, and minimize jerk. I used a non-linear optimizer (Ipopt) and massaged the code I wrote for model-predictive control. Things worked out quite nicely.
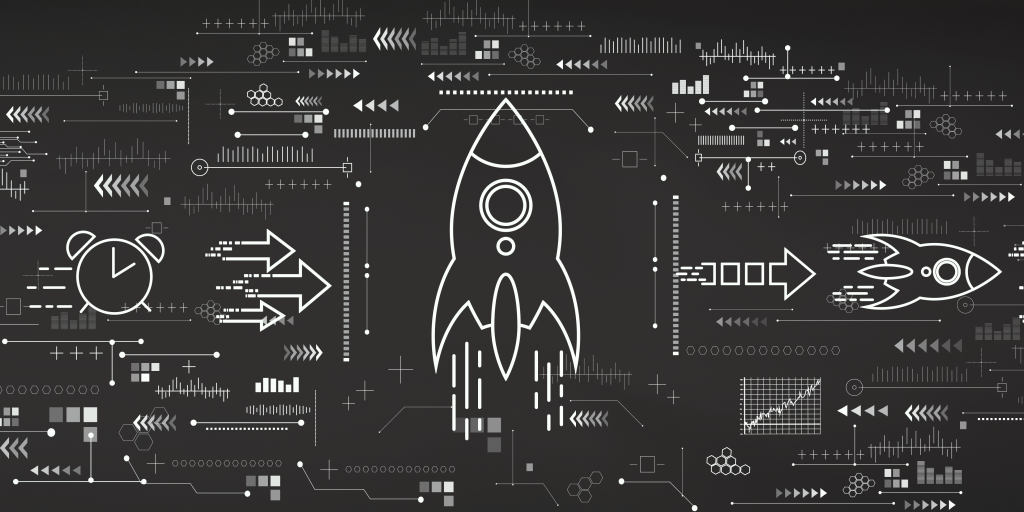
I have recently stumbled upon two articles on running TensorFlow on CPU setups and decided to check how well that works for the models I use. The results were somewhat unexpected.
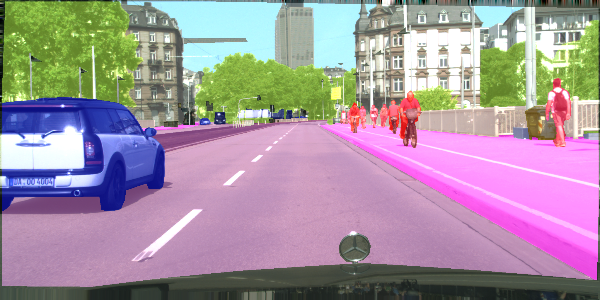
Semantic segmentation is a process of dividing an image into sets of pixels sharing similar properties and assigning one of the pre-defined labels to each of these sets. Or, in other words, you get a picture, and you're supposed to tell which pixels constitute a car and which constitute the pedestrians. Fun stuff.
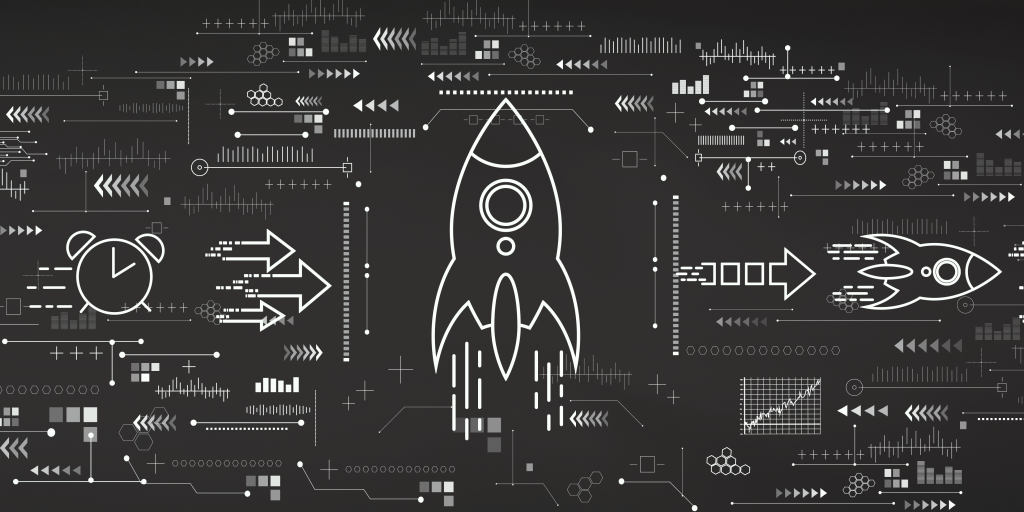
This post is an update to the previous post discussing a newer version of TensorFlow.
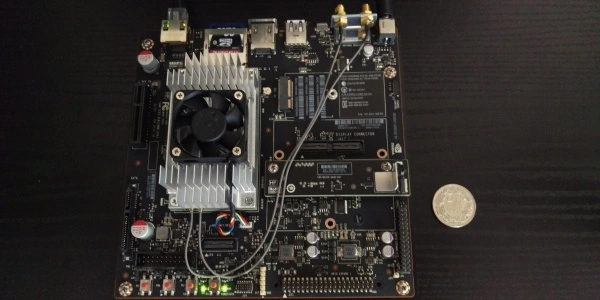
I need to run my neural network models on this board, so I need TensorFlow to run on it. I had expected a smooth ride, but it turned out to be quite an adventure and not one of a pleasant kind. Here's a how-to, so you don't have to waste time figuring it out yourself.
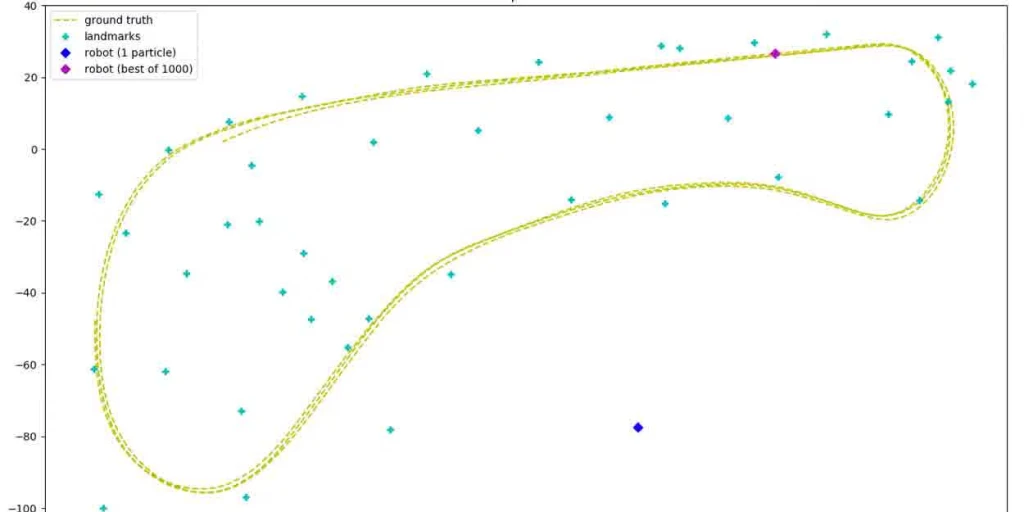
Playing with particle filters. They are a cool way to estimate a robot's position based on noisy signals and using randomness.
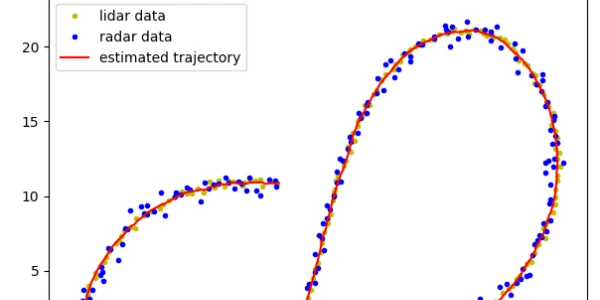
Estimating a trajectory of an object using Kalman filters based on radar and lidar data.
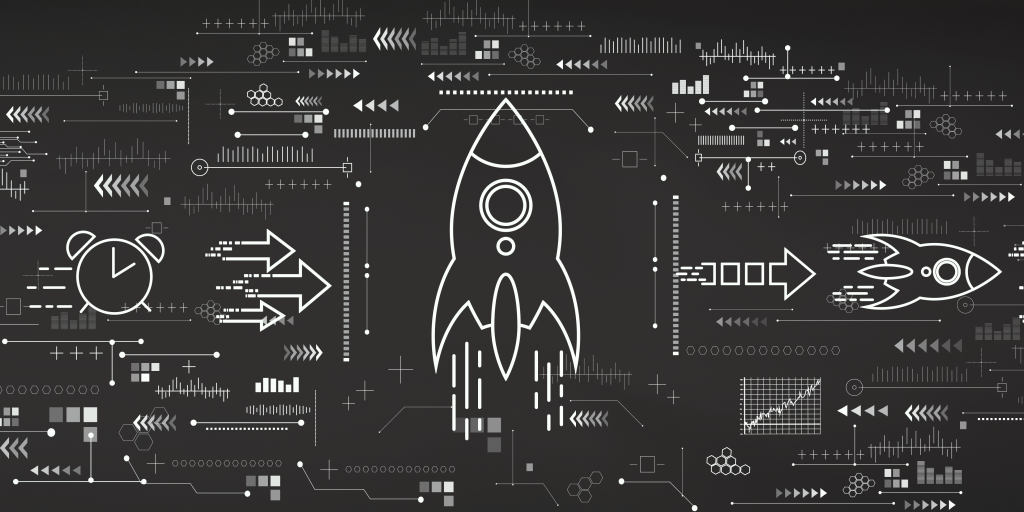
I have a love-hate relationship with developing software for embedded systems. On the one hand, it's loads of fun. On the other, there seem to be lots of closed-up magic proprietary blobs required to make things work. The same can usually be achieved with pure open source if you put some time and understanding into it. This post describes an open-source template for FRDM-K64F.
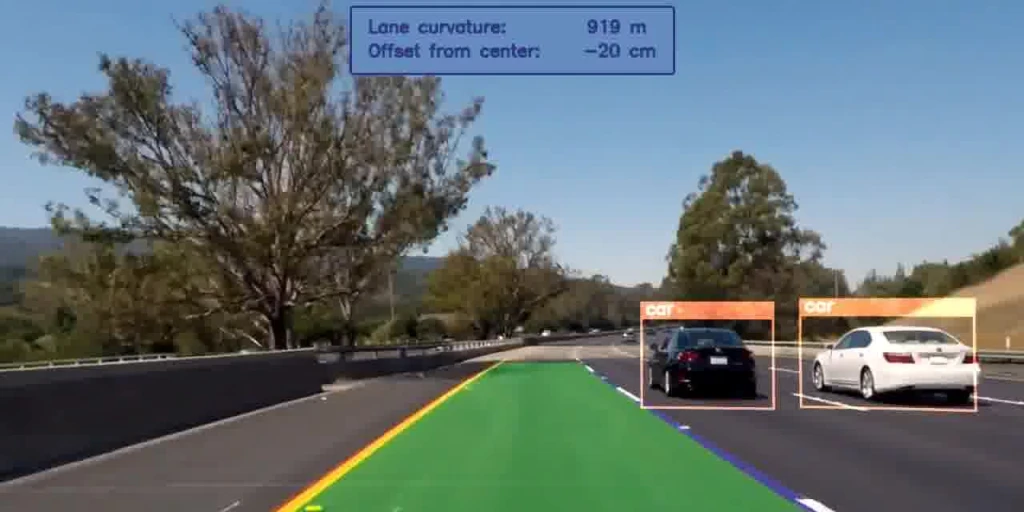
In this post, I am playing with some classical computer vision algorithms and Support Vector Machines to see where the lane lines and other vehicles are in a video taken by a front-facing camera in a car driving on a highway.
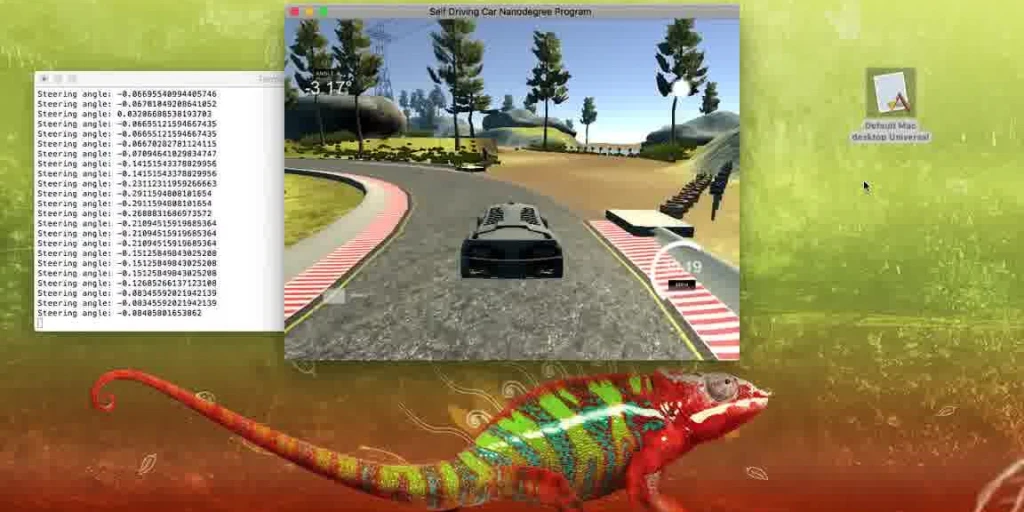
You get to drive a car in a game capturing some images and the corresponding steering angle. You then use that data to build a neural network that spits out a steering angle for an input image such that the computer can drive the same car in the same game. The computer learns how to drive from you, hence behavioral cloning.