Content tagged tensorflow
Tags
Months
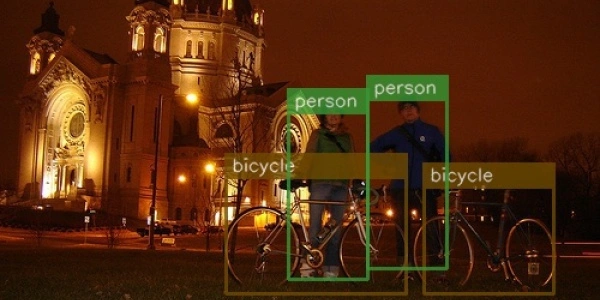
Some time ago, I wrote a post on building TensorFlow on a Jetson TX1. It worked eventually, but it was relatively slow because it's an edge device and it's not very powerful. It's way faster to compile it on a PC and copy the binaries to the target board. Here's how.
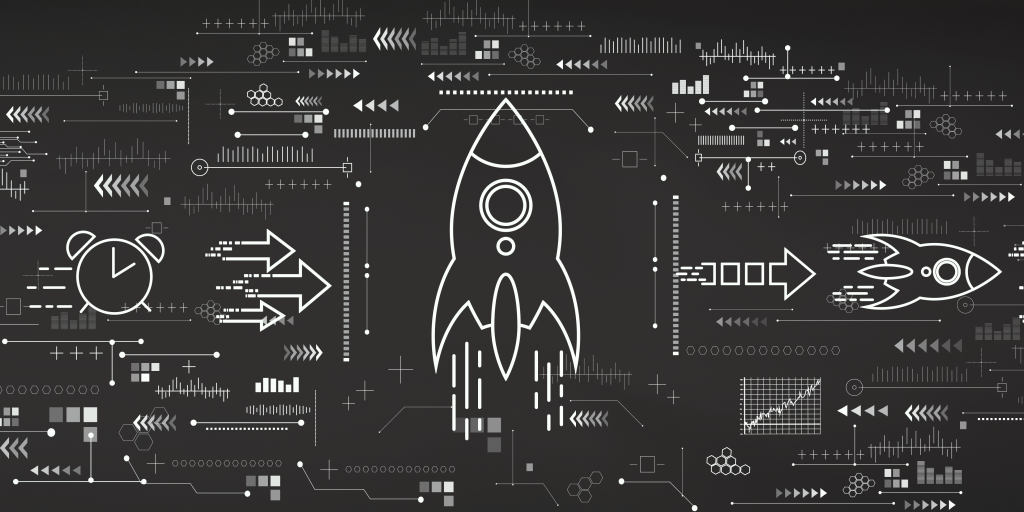
AWS has recently introduced the P3 instances. They come with Tesla V100 GPUs, so I decided to run a little benchmark to see how well they perform compared to my workstation (GeForce 1080 Ti) when training neural networks.
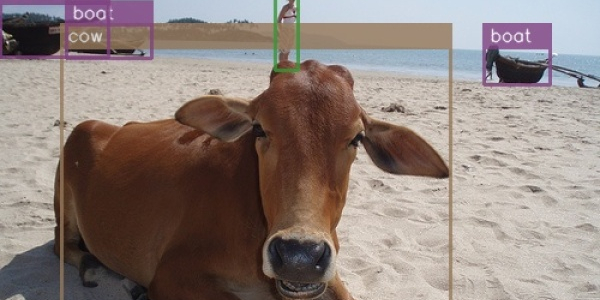
There was no understandable and straightforward implementation of SSD in TensorFlow, so I decided to make one. The original paper assumes familiarity with related research, so I needed to plow through several additional papers and a ton of source code to understand what's going on here. This post is an attempt to provide all that missing context in one place.
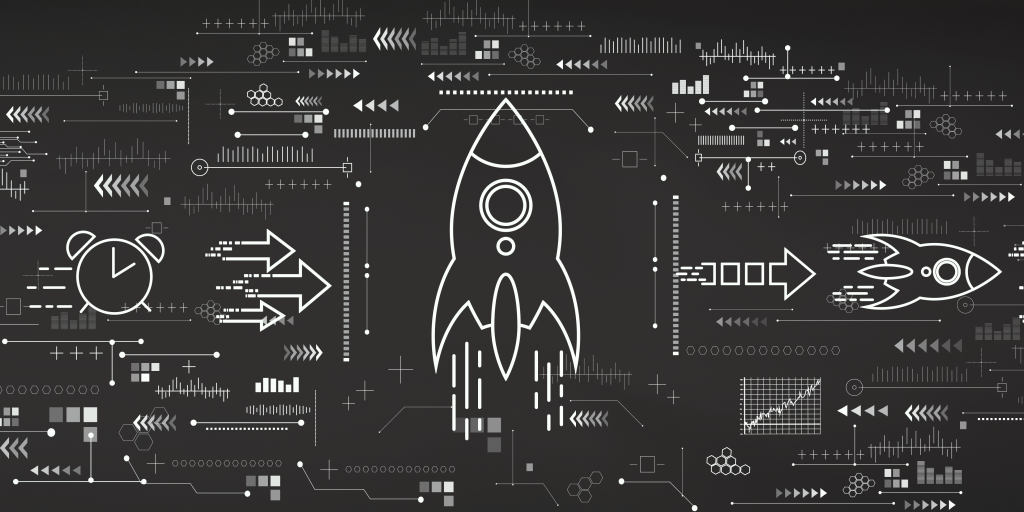
I have recently stumbled upon two articles on running TensorFlow on CPU setups and decided to check how well that works for the models I use. The results were somewhat unexpected.
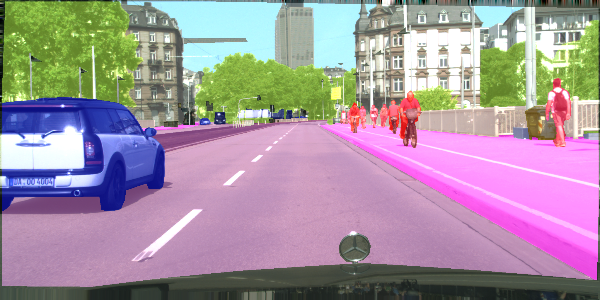
Semantic segmentation is a process of dividing an image into sets of pixels sharing similar properties and assigning one of the pre-defined labels to each of these sets. Or, in other words, you get a picture, and you're supposed to tell which pixels constitute a car and which constitute the pedestrians. Fun stuff.
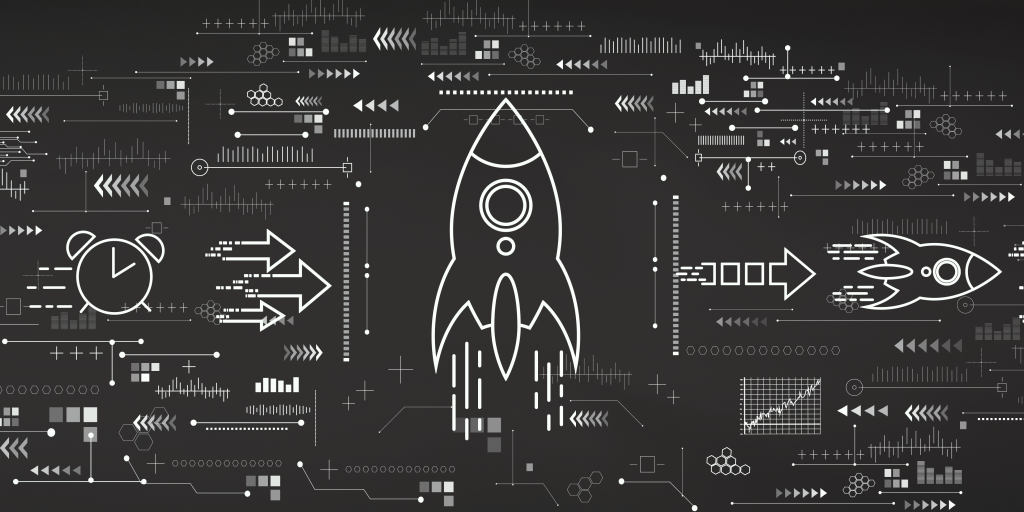
This post is an update to the previous post discussing a newer version of TensorFlow.
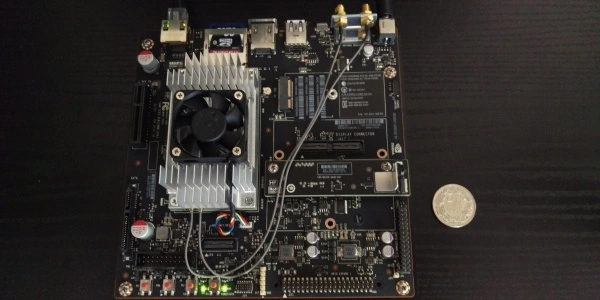
I need to run my neural network models on this board, so I need TensorFlow to run on it. I had expected a smooth ride, but it turned out to be quite an adventure and not one of a pleasant kind. Here's a how-to, so you don't have to waste time figuring it out yourself.